Data Strategy for AI Success: Building Your Foundation
A structured approach to developing and executing a data strategy that enables AI success and drives business value for organizations of all sizes.

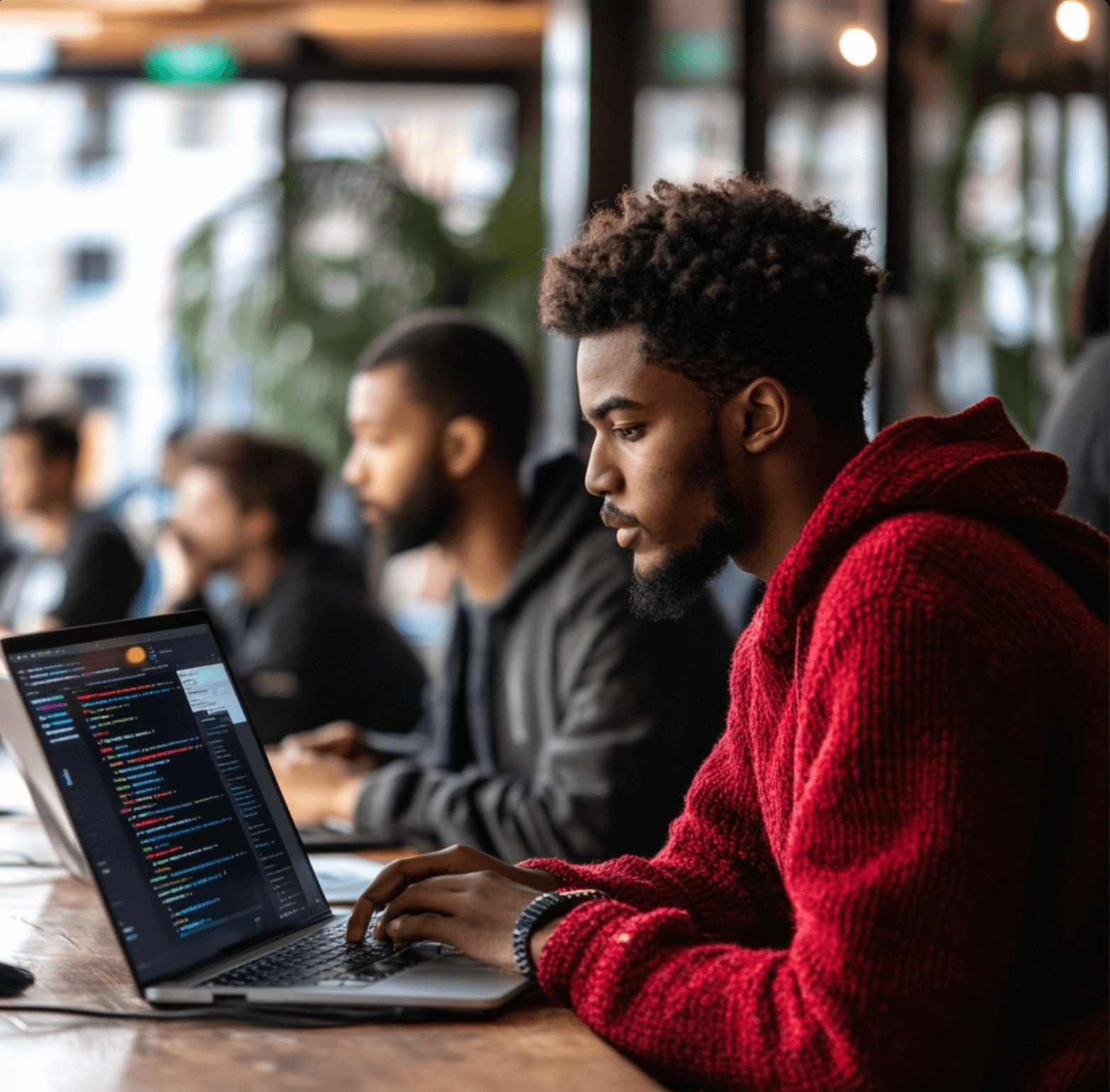
A robust data strategy is essential for successful artificial intelligence implementation. This guide provides business leaders with a structured approach to developing and executing a data strategy that enables AI success and drives business value.
Understanding Data's Critical Role in AI Success
Data is the foundation of effective AI systems, determining the quality, accuracy, and value of AI outputs. According to McKinsey's research, organizations have been experimenting with generative AI tools, but from a value capture standpoint, these are still early days—few are experiencing meaningful bottom-line impacts. This gap between adoption and value often stems from inadequate data strategies.
A comprehensive data strategy enables:
- High-quality training data for AI models
- Accessible and usable data across the organization
- Appropriate governance and compliance practices
- Integration of diverse data sources for richer insights
- Continuous data quality improvement
Recent research indicates that data privacy in AI is the biggest challenge the technology faces, with concerns about algorithm bias, privacy risks, transparency ethics, and technology regulation. This highlights the importance of addressing these aspects in your data strategy.
Strategic Data Assessment
Begin by thoroughly evaluating your current data landscape:
Data Inventory and Mapping
- Catalog existing data assets across the organization
- Document data sources, types, and formats
- Map data flows between systems
- Identify data owners and stewards
- Assess regulatory and compliance considerations
Quality and Usability Evaluation
- Evaluate data completeness and accuracy
- Assess data accessibility and integration capability
- Review data standardization and consistency
- Identify data gaps relevant to AI initiatives
- Determine data freshness and update frequency
Governance and Compliance Review
- Assess current data governance structures
- Review privacy policies and practices
- Evaluate security measures and protections
- Identify compliance issues and risks
- Review data ethics considerations
AI Readiness Analysis
- Determine suitability of data for AI applications
- Assess data volumes and diversity
- Evaluate data annotation and labeling needs
- Identify bias potentials and mitigation options
- Determine computational and storage requirements
This assessment provides the foundation for targeted strategic initiatives, helping organizations understand their starting point and key focus areas.
Data Strategy Framework
Develop a comprehensive data strategy with these key components:
Vision and Objectives
- Define clear data vision aligned with business strategy
- Establish specific, measurable objectives
- Create linkage to AI and business goals
- Develop value-based prioritization framework
- Set timeline and milestones for implementation
Governance and Organization
- Design data governance structure and roles
- Establish decision rights and responsibilities
- Define data policies and standards
- Create data quality management processes
- Develop monitoring and compliance mechanisms
Architecture and Infrastructure
- Design appropriate data architecture
- Plan necessary infrastructure improvements
- Determine cloud vs. on-premises approaches
- Establish integration frameworks and methods
- Plan for scalability and performance
Data Management and Operations
- Define data lifecycle management processes
- Establish metadata management approaches
- Create data quality improvement initiatives
- Develop master data management strategies
- Design data operations and support model
By addressing each of these components, organizations create a comprehensive approach that enables effective AI deployment while managing risks appropriately.
Building Your Data Foundation for AI
Focus on these initiatives to create a strong data foundation:
Data Collection and Integration
- Implement systematic data collection processes
- Create unified data access mechanisms
- Develop API and integration strategies
- Establish real-time data capabilities where needed
- Build data pipelines for AI applications
Quality Enhancement and Preparation
- Implement data cleaning and standardization
- Develop validation rules and processes
- Create enrichment and augmentation capabilities
- Establish testing and verification protocols
- Build annotation and labeling capabilities
Governance Implementation
- Roll out governance structures and processes
- Implement data catalogs and dictionaries
- Establish data stewardship practices
- Deploy privacy and security controls
- Create audit and compliance mechanisms
AI-Specific Data Preparation
- Develop training data sets for AI models
- Create testing and validation data
- Implement bias detection and mitigation
- Establish feedback loops for continuous improvement
- Build data versioning and lineage tracking
Recent findings highlight that AI spending in the manufacturing sector is projected to reach $15.7 billion by 2025, demonstrating significant investment in data-driven AI capabilities.
Data Governance for AI
Implement these governance practices specific to AI applications:
Ethical Data Usage
- Establish principles for responsible data use
- Create review processes for AI training data
- Implement bias detection and mitigation
- Develop transparency and explainability standards
- Establish accountability for data decisions
Privacy Protection
- Implement privacy by design practices
- Develop data minimization approaches
- Create anonymization and de-identification capabilities
- Establish consent management processes
- Deploy privacy-enhancing technologies
Security and Access Control
- Implement appropriate security controls
- Create risk-based access management
- Develop monitoring and alerting systems
- Establish incident response procedures
- Build security awareness and training
Regulatory Compliance
- Develop compliance frameworks for relevant regulations
- Create documentation and evidence collection
- Establish ongoing monitoring and assessment
- Implement compliance by design practices
- Build regulatory change management processes
Effective governance ensures that data usage aligns with ethical standards and regulatory requirements while enabling innovation and value creation.
Organizational Capabilities and Culture
Develop these essential capabilities for data strategy success:
Leadership and Sponsorship
- Secure executive commitment to data strategy
- Establish clear accountability for implementation
- Align incentives with data strategy objectives
- Provide necessary resources and investment
- Create visible leadership engagement
Talent and Skills Development
- Assess current data-related capabilities
- Identify skill gaps and development needs
- Create training and educational programs
- Recruit specialized talent where necessary
- Develop communities of practice
Data Literacy and Culture
- Build organization-wide data literacy
- Create data-driven decision-making processes
- Establish data sharing and collaboration norms
- Develop recognition for data excellence
- Implement change management for data practices
External Ecosystem Engagement
- Identify potential data partnership opportunities
- Explore industry data sharing initiatives
- Engage with technology and service providers
- Participate in relevant standards bodies
- Connect with academic and research organizations
These capabilities ensure that the organization can execute the data strategy effectively and derive maximum value from its data assets.
Implementation Roadmap
Follow this phased approach to data strategy implementation:
Phase 1: Foundation Building
- Establish governance structures and roles
- Implement initial policies and standards
- Deploy basic data catalog and inventory
- Address critical quality and compliance issues
- Build initial data platforms and infrastructure
Phase 2: Capability Development
- Implement data quality management processes
- Deploy master data management capabilities
- Build advanced data integration features
- Develop specialized AI data capabilities
- Create data literacy and training programs
Phase 3: Advanced Enablement
- Implement advanced analytics environments
- Deploy sophisticated governance tools
- Create specialized AI data pipelines
- Implement automated quality management
- Develop self-service capabilities
Phase 4: Innovation and Optimization
- Implement cutting-edge data technologies
- Explore advanced data monetization
- Create cross-enterprise data capabilities
- Develop ecosystem data exchange
- Optimize for continued performance improvement
This phased approach allows organizations to build capabilities progressively while delivering incremental value throughout the implementation journey.
Measuring Data Strategy Success
Track these metrics to evaluate your data strategy effectiveness:
Data Quality Metrics
- Completeness and accuracy rates
- Consistency across systems
- Timeliness and currency
- Duplication and redundancy
- Quality issue resolution time
Operational Metrics
- Data access and retrieval time
- Integration efficiency and reliability
- Process automation levels
- Governance compliance rates
- Incident and issue frequency
Business Impact Metrics
- AI model performance improvement
- Decision-making speed and quality
- Cost reduction and efficiency gains
- Revenue and profit impact
- Competitive advantage creation
Strategic Capability Metrics
- Data literacy levels
- Talent attraction and retention
- Innovation capability improvement
- Partner engagement effectiveness
- Technology adoption and utilization
Comprehensive measurement ensures that data investments deliver tangible business value while building long-term capabilities.
Common Challenges and Mitigation Strategies
Be prepared to address these common challenges:
Legacy Systems and Technical Debt
- Challenge: Outdated systems with limited data capabilities
- Solution: Modernization roadmap with interim integration
- Implementation: Phased approach prioritizing highest-value systems
Organizational Silos
- Challenge: Fragmented data ownership and resistance to sharing
- Solution: Cross-functional governance and incentive alignment
- Implementation: Executive-sponsored data sharing initiatives
Talent and Skill Gaps
- Challenge: Insufficient specialized data skills
- Solution: Combination of hiring, training, and partnerships
- Implementation: Prioritize critical roles while building internal capability
Regulatory Complexity
- Challenge: Evolving and complex data regulations
- Solution: Comprehensive compliance framework with monitoring
- Implementation: Privacy and compliance by design in all initiatives
By proactively addressing these challenges, organizations can accelerate their data strategy implementation and value realization.
Looking Forward: Emerging Data Trends
Prepare for these emerging developments in data strategy:
Federated Data Approaches
- Data remains distributed but accessible
- Local processing with global insights
- Enhanced privacy protection
- Reduced data movement and duplication
- Simplified governance and compliance
Data Mesh Architecture
- Domain-oriented data ownership
- Data as a product mindset
- Self-serve data infrastructure
- Federated computational governance
- Distributed data generation and consumption
AI-Driven Data Management
- Automated data quality management
- AI-powered metadata generation
- Intelligent data discovery and classification
- Automated compliance monitoring
- Self-optimizing data systems
By establishing a comprehensive data strategy with these elements, organizations create the foundation for successful AI implementation and sustainable competitive advantage through data-driven intelligence and decision-making.
About the Author
Related Articles
Subscribe to Our Newsletter
Get the latest insights on AI innovation, content creation, and career advancement delivered to your inbox.